How AI is shaping app interactivity.
As the dust settles from the annual soul-bearing digital event we’ve come to love and fear in equal measures, Spotify Wrapped is a beautiful example of a web and mobile app that’s leveraging data analytics, machine learning (ML), and artificial intelligence (AI) to create personalised, interactive user experiences. Whether you’re blaming your kids for your George Ezra-dominated top tracks, proudly declaring your “Swiftie” status or fervently googling the definition of “crank wave”, one thing’s clear: you’re not keeping your Wrapped screenshots to yourself.
In recent years, the angry “they’re listening” complaints during app usage have begun to dissipate with the value exchange consumers are experiencing from brands leveraging large datasets and machine learning algorithms effectively and – more importantly – ethically.
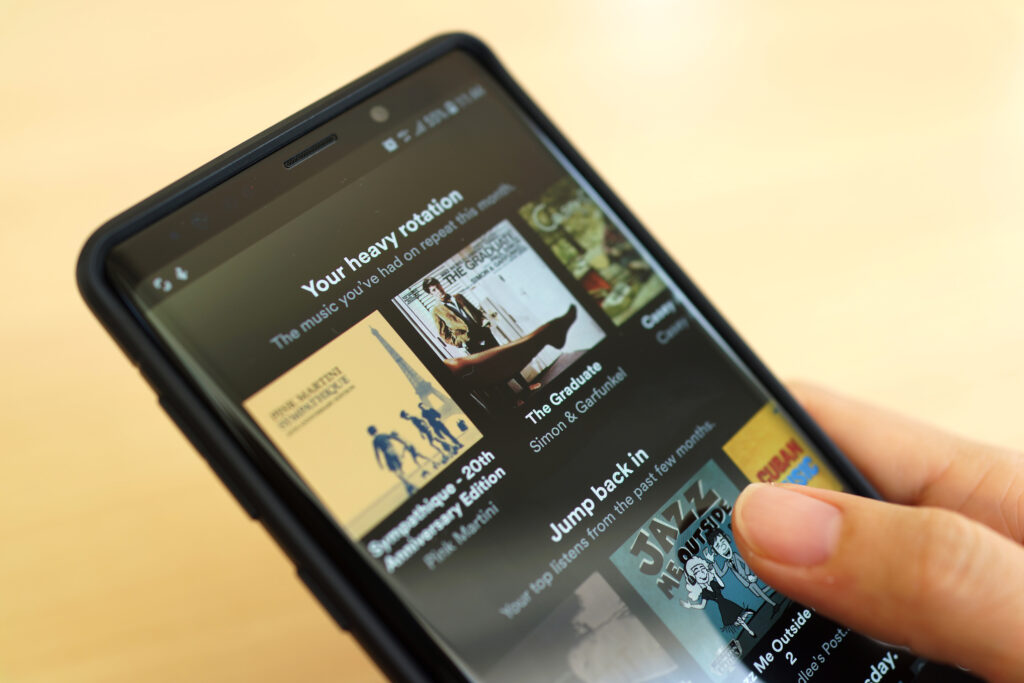
Companies can learn a lot from Spotify’s handling of app user habits, not least its exemplary compilation and presentation of their vast amount of data into visually striking, shareworthy content that has also been harnessed to create multiple user opportunities, including artist engagement, bespoke merchandise and recommended events. Crucially, 2023 is also the first year that Spotify Wrapped can be navigated using a screen reader – meaning that blind and partially-sighted people can also take part, making the app as awesome as it is accessible.
In a new dawn of interactivity and intelligence, web app development is traversing a transformative shift into the realm of AI and ML to revolutionise user experiences like never before. And, while concerns about data privacy persist, consumers are increasingly willing to part with their precious data if they’re to be rewarded with highly tangible and valuable brand experiences – particularly when they have control over their privacy settings.
The rise of personalised app experiences.
The distaste for personalised ads and creepy omniscient recommendations is a far cry from the hyper-tailored brand experiences we’ve come to expect.
The entertainment industry has trailblazed in popularising personalised content. Netflix’s recommendation system is a prime example. Its algorithms analyse viewing habits (yep, they know you’re a binger), preferences (and all about your morbid fascination with serial killers), and interactions to provide personalised suggestions in the ‘Recommended for you’ section, which has a significant influence over what its users watch, while driving engagement and retention. YouTube’s recommendation algorithm follows a similar format, suggesting videos based on watch history, likes, and interactions. This influences user behaviour by keeping people engaged for longer periods, leading to increased viewing time and ad revenue.
In hospitality, Airbnb’s user experience has consistently out-done itself. When it comes to recommendations, the app suggests personalised accommodation options based on past searches, preferences, and previous bookings. This tailored and creative approach influences booking decisions, encouraging users to plan stays aligned with their interests and past experiences, as well as experiment beyond the typical booking parameters (a Pembrokeshire weekend in a UFO ‘futuro styled flying saucer’ is the trip you didn’t know you needed).
Over in retail, Sephora’s use of AI-powered personalised beauty recommendations helps customers find products suited to their preferences and skin types. By analysing customer data and providing tailored product suggestions, Sephora drives sales and enhances user satisfaction.
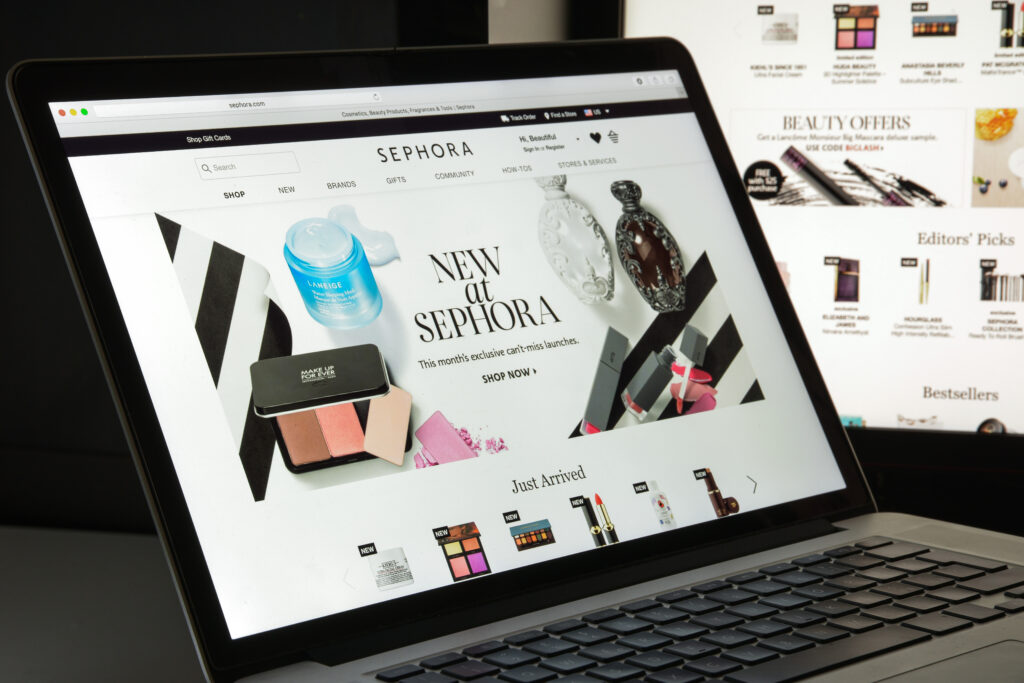
In the financial world, the Albert mobile banking app uses AI, ML and data analysis to analyse and forecast users’ financial situations – including income, expenses, and spending patterns – to provide personalised insights and recommendations such as optimised spending suggestions, identified areas for saving, or bespoke investment advice.
“From the customer experience perspective, AI and ML are playing pivotal roles in personalising user experiences. AI algorithms can analyse spending patterns and preferences, leading to tailored rewards and offers. Imagine a credit card that not only rewards you for purchases but also suggests where you might want to use your points based on your past behaviour. It’s like having a personal financial advisor embedded in your card.”
Sarat Pediredla, hedgehog lab CEO and Co-Founder
Over in the health industry, Bupa’s AI-powered mental health service that’s offered through its Blua Health app allows users to:
- Book 24/7 GP appointments
- Access personalised healthcare insights
- Get support with Symptom Checker
- Order and track prescriptions
Data points such as a daily questionnaire plus exercise and sleep data, are gathered through wearable devices to analyse the user’s mental health. It uses AI to provide the customer with personalised content for managing their mental health and even offers therapist appointments if the customer’s mental health is consistently poor. This service has been proven to detect early signs of mental health conditions – a crucial and highly valuable tool given that, according to mental health charity Mind, 1 in 4 people will experience a mental health problem of some kind each year in England.
What each of these brands has in common is the ability to leverage AI, data, and machine learning to create truly delightful and meaningful user experiences. By analysing user data, preferences and behaviour, these platforms consistently deliver highly personalised recommendations and profound interactions.
Shifting consumer perceptions in ux/ui design.
There’s been a noticeable shift in consumer mentality and perception when it comes to data sharing and personalised content. Initially (and understandably), there was significant security scepticism and privacy concerns surrounding the sharing of personal data. But, over time, several factors have influenced this change:
- Value exchange: Consumers are more willing to share data when they see a clear value in return. In response, savvy brands have shifted their approach from merely collecting data to providing tangible benefits in exchange. Personalised content, relevant recommendations, exclusive offers, preference-tailored interfaces and an all-around more engaging and interactive user experiences are all considered valuable by users. According to Statista, ‘In 2023, around three-quarters of surveyed global customers expressed their expectation for improved personalisation when sharing more personal data with businesses.
- Transparency and trust: To gain and maintain consumer trust, brands have become increasingly transparent about how they collect, store, and use data. This includes clear privacy policies, easy-to-understand terms and user-friendly interfaces (queue animation of a cute animal consuming ‘cookies’) enabling consumers to easily control their data preferences. Brands have also taken steps to educate users about data usage, communicating the benefits of sharing data and how it improves user experiences.
- Empowerment through control: Rightly so, users demand control over their data. To harness customer insights and build more personalised brand experiences, companies need to provide more robust privacy settings, allowing users to adjust preferences, easily opt out of certain data collection, or even delete their data. This sense of control empowers users to share their data on their terms, in turn helping to build brand trust.
- Ethical data usage: With enhanced security measures and strict data protection regulations like GDPR, companies are forced to adopt ethical data practices, ensuring that collected data is used solely for stated purposes and that user preferences are respected. As a result, consumers are more comfortable that their data is being handled responsibly, and trust surrounding data-sharing practices is steadily being restored.
- The prevalence of personalisation: As personalised content becomes more prevalent across various platforms, consumers have become not only accustomed to but expectant of high-value personalised experiences – particularly when they’re paying top dollar for products and services in an economy that isn’t exactly encouraging frivolous spending. According to Statista, ‘73 per cent [of surveyed global customers] indicated their anticipation of a more personalised experience as technology advances, and 64 per cent expected the same when increasing their spending.’
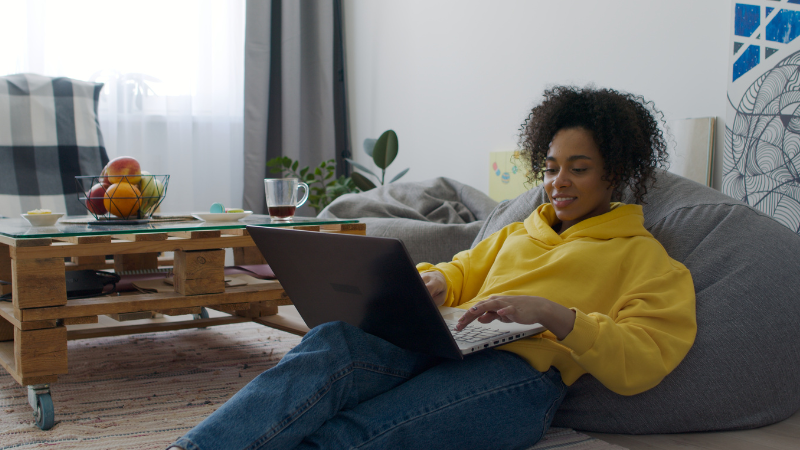
Machine learning strategies for web and mobile apps.
The web and mobile apps of the future are so much more than the (digitally) dust-gathering platforms that used to sit on our phones, sporadically used, sapping valuable storage space. Apps have become intelligent beyond belief, intelligently and intuitively integrating with our day-to-day lives and making a significant difference to the way we approach purchasing, learning, health, wellbeing and socialising.
As consumer expectations soar to new heights of personalisation, the future of these apps hinges on their ability to harness AI and ML to decode user behaviours, predict pain points, deliver value, and curate interactive user experiences that are not just adaptive, but perceptive to the subtlest human needs and nuances.
However, as inspiring a case study as Spotify is, it goes without saying that it’s no ordinary enterprise. To cope with an excess of 1 trillion events being captured every day, the Spotify Engineering team is responsible for 2,900+ deployments per day, 34,000+ data pipelines, 3,000+ engineers and 3,200+ microservices in production.
So, we can comfortably conclude that not only is their data engineering team much bigger than most businesses, but their IT budget is also significantly larger. That means Spotify has the resources to acquire, deploy, and customise its data engineering for a large number of transformative solutions tailored to its vast infrastructure.
For most businesses looking to achieve personalised, interactive mobile and web app experiences by integrating machine learning into its processes, an achievable solution would involve the following steps:
Define objectives and use cases.
- Identify specific areas where personalisation can significantly impact your user experience, such as content recommendations, user segmentation and adaptive interfaces.
Data collection and preparation.
- Gather and organise relevant data from web and app interactions, user profiles, preferences, and behaviours.
- Ensure data quality and consistency.
- Implement data pipelines and workflows to preprocess and clean the data, making it ready for machine learning analysis.
Choose appropriate machine learning models.
- Select machine learning algorithms suitable for your use cases. For instance, collaborative filtering for recommendation systems, clustering for user segmentation, or natural language processing for text-based interactions.
- Leverage pre-built models or frameworks (like scikit-learn, TensorFlow, or PyTorch) to expedite model development.
Feature engineering for personalisation.
- Engineer relevant features from the collected data that can enhance personalisation and interactivity. For example, user behaviour sequences, time-based patterns, or location-based preferences.
Model development and testing.
- Develop machine learning models based on the selected algorithms and features. Train and test these models using historical data to ensure accuracy and effectiveness.
- Use A/B testing or split testing to evaluate model performance against different user groups or scenarios before full deployment.
Integration into web and mobile apps.
- Integrate the trained models into the web and mobile app infrastructure. Ensure they can handle real-time or near-real-time interactions for personalised recommendations or adaptive interfaces.
Continuous monitoring and improvement.
- Implement monitoring systems to track model performance and user interactions. Continuously collect feedback and data to refine and improve the models over time.
- Use techniques like reinforcement learning to allow models to adapt and improve based on ongoing user interactions.
User engagement and communication.
- Communicate the personalised features to your users transparently.
- Empower users with control over their data and personalisation settings, ensuring compliance with privacy regulations.
Cross-functional collaboration.
- Foster collaboration between data scientists, engineers, UX/UI designers, and product managers to align the machine learning efforts with user experience goals.
If you’d like to discuss how to boost your web or mobile app’s interactivity and personalisation capabilities, get in touch or explore our UX/UI design services and AI packages.